The Pre-Doctoral Training Program in Computational Medicine commences the 2018-19 academic year with a cohort of six PhD students, an increase from last year’s inaugural cohort of four students.
The Program, funded by the National Institute of General Medical Sciences under Award Number T32GM119998, is the first of its kind and provides 12 months of support for selected PhD students from the departments of Biomedical Engineering and Applied Mathematics & Statistics. Students chosen for this Ruth L. Kirschstein National Research Service Award institutional training grant learn through a combination of focused coursework and dissertation research alongside computational medicine training program faculty mentors from across the Johns Hopkins Whiting School of Engineering and the School of Medicine.
Thomas Athey, Adriana Gonzalez, Ashton Omdahl, and Markus Sommer, first year PhD students in the department of biomedical engineering; Jason Miller, a second year PhD student in the department of applied mathematics and statistics; and Dan Popescu, a third year PhD student in the department of applied mathematics and statistics, comprise the 2018-19 cohort.
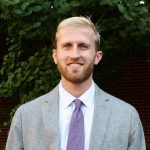
THOMAS ATHEY
A Johns Hopkins alumnus, Thomas Athey received a Bachelor of Science in Biomedical Engineering and a Minor in Computational Medicine in 2018. As an undergraduate, Athey worked in the Institute for Computational Medicine laboratories of Michael I. Miller, director of the Department of Biomedical Engineering, and Tilak Ratnanather, associate research professor of biomedical engineering. In May 2018, Athey presented his research at ICM’s annual Computational Medicine Night.
Athey explains his interest in the discipline of computational medicine, “I believe that computational tools uniquely accommodate the multi-scale and nonlinear nature of the human body’s response to medicine. Computational medicine is an exemplary engineering discipline because it calls for cutting edge theoretical methods for the purpose of tangible medical results.”
Athey’s primary research interest is automated image interpretation. He appreciates the data science challenges associated with medical applications such as small sample size, feature dependence, and class bias.
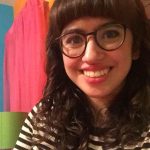
ADRIANA GONZALEZ
Adriana Gonzalez graduated from New York University in 2017 where she earned a Bachelor of Science in Biology. During the summers of 2013 and 2014, Gonzalez was a visiting undergraduate researcher at Johns Hopkins in the laboratory of Feilim Mac Gabhann, associate professor of biomedical engineering, ICM faculty member, and co-director of the Pre-Doctoral Training Program in Computational Medicine.
Data and the possibility of demystifying it is what attracted Gonzalez to computational medicine. Genetics and genomics were her favorite parts of biology.
“I was entranced by how the seemingly simple codes of DNA and RNA gave rise to such complicated systems and organisms,” says Gonzalez. “Going from the code to a person, there are so many ways things can go wrong, and I am interested in unraveling the interactions all along that path with the aim of improving human health,” she adds.
Broadly, Gonzalez is interested in applying statistical methods for analyzing gene expression patterns in disease. Her past research involved analyzing VEGF family gene expression in colon cancer, and teasing out potential therapeutic targets from seemingly meaningless piles of data. Gonzalez has also worked with microbiome data associated with rheumatoid arthritis, which is particularly exciting to her because of its complexity.
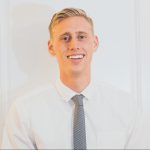
ASHTON OMDAHL
Ashton Omdahl was interested in biology from an early age and developed an interest in computational modeling tools for drug discovery while participating in Science Olympiad in high school. The experience opened his mind to the possibilities of computational modeling and the problem solving nature of computer science. In 2018 Omdahl earned a Bachelor of Science in Bioinformatics from Brigham Young University.
Omdahl was attracted to computational medicine for its ability to synthesize his academic interests in a way that directly impacts the lives of people. He is pursuing research in bioinformatics methods and their applications.
Omdahl’s research experience also includes gene co-expression analysis, on which he comments, “I am excited to see how this kind of work could be applied to personalize patient care and target drug treatments to maximize efficacy while minimizing side effects.”
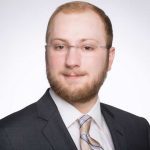
MARKUS SOMMER
Markus Sommer completed a Bachelor of Science in Molecular Biology at The University of California, Berkeley in 2015 and worked as a researcher in the Departments of Radiology and Biomedical Engineering at The University of California, San Francisco from 2015 to 2018 before pursuing his PhD.
Sommer sees the Training Program in Computational Medicine as an opportunity to combine his passion for quantitative research with his desire to make an impact on the lives of those around him.
“Working within the vibrant biomedical engineering community at Johns Hopkins, I hope to harness the incredible resources available to students to help expose and attack the underlying mechanisms of disease,” explains Sommer.
Sommer believes that genomic research provides a unique opportunity to bring powerful machine learning tools to bear on the most fundamental human data: DNA. He is interested in translating modern techniques from computer science to genomics, forging new ways to understand and improve the human condition.
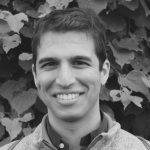
JASON MILLER
Jason Miller attended The University of Virginia where he earned a Bachelor of Science and a Masters in Mathematics in 2014 and 2015, respectively. Miller worked as a quantitative research analyst for Strategic Investment Group from 2015 to 2017 before entering the PhD program in the Department of Applied Mathematics and Statistics.
Miller’s interest in computational medicine was driven by the opportunity to apply diverse mathematics, ranging from harmonic analysis and signal processing to dynamical systems and machine learning, to solve problems that have impact in the clinic for patients.
“Developing new machine learning and optimization algorithms that are interesting from a mathematical perspective and can handle the profound challenges posed by medical data in its various high-dimensional forms is a major research goal of mine,” Miller explains.
Miller’s research involves applying wavelets and multi-scale analysis to model the regularity and complexity of scar tissue within the heart, then applying machine learning to create a fast and predictive model for patients’ risk of sudden cardiac death based on their scar tissue patterns.
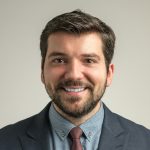
DAN POPESCU
Dan Popescu graduated from the University of Richmond in 2012 with a Bachelor of Science in Mathematics and Economics and was employed by Goldman Sachs as a strategist from 2012 to 2016 prior to starting the PhD program in Applied Mathematics and Statistics.
Popescu describes his affinity for the discipline of computational medicine, “Computational medicine is a relatively new field which provides ample opportunities to study problems with real-world impact. It is one of the few fields where the road from theory to practice is very short, making it a really rewarding field in which to research.”
Popescu’s interest is applying mathematics to solving problems stemming from science. His current research focuses on machine learning techniques for predicting risk of various cardiovascular events in the ICM laboratory of Natalia Trayanova, Murray B. Sachs Professor in the Department of Biomedical Engineering. Given the vast data sets of medical images available at Johns Hopkins, this area of focus harmoniously combines Popescu’s passion for machine learning and computer vision.
The Pre-Doctoral Training Program in Computational Medicine provides participants with unique experiences in the field of computational medicine in their year of support and beyond. In addition to completing coursework, the trainees become part of the Institute for Computational Medicine’ s scientific community by participating in a computational medicine journal club, attending ICM seminars and the annual ICM retreat, working with clinicians, and planning a student-run computational medicine conference.
Upon completion of the program, trainees will receive PhD degrees from their home department as well as research experience and training designed to prepare graduates to fill the growing need for researchers trained in computational medicine in both industry and academia.