Bio
“Big Data Approaches to Study
Intercellular Signaling during
Tumor Immune Evasion”
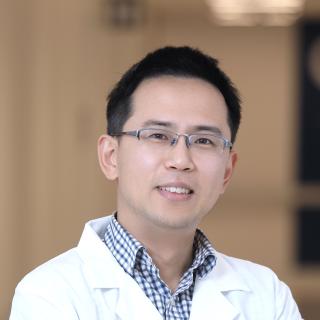
Dr. Peng Jiang started his research program at the National Cancer Institute (NCI) in July 2019. His Lab focuses on developing big-data and artificial intelligence frameworks to identify biomarkers and new therapeutic approaches for cancer immunotherapies in solid tumors. Before joining NCI, he finished his postdoctoral training at the Dana Farber Cancer Institute and Harvard University. During his postdoctoral research, Peng developed computational frameworks that repurposed public domain data to identify biomarkers and regulators of cancer immunotherapy resistance. Notably, his computational model TIDE revealed that cancer cells could utilize the self-protection strategy of cytotoxic lymphocytes to resist lymphocyte killing under immune checkpoint blockade. Dr. Peng finished his Ph.D. at the Department of Computer Science & Lewis Sigler Genomics Institute at Princeton University, and his undergraduate study with the highest national honors at the Department of Computer Science at Tsinghua University (GPA rank 1st in his year). He is a recipient of the NCI K99 Pathway to Independence Award, the Scholar-In-Training Award of the American Association of Cancer Research, and the Technology Innovation Award of the Cancer Research Institute.
▶RECORDING
Abstract
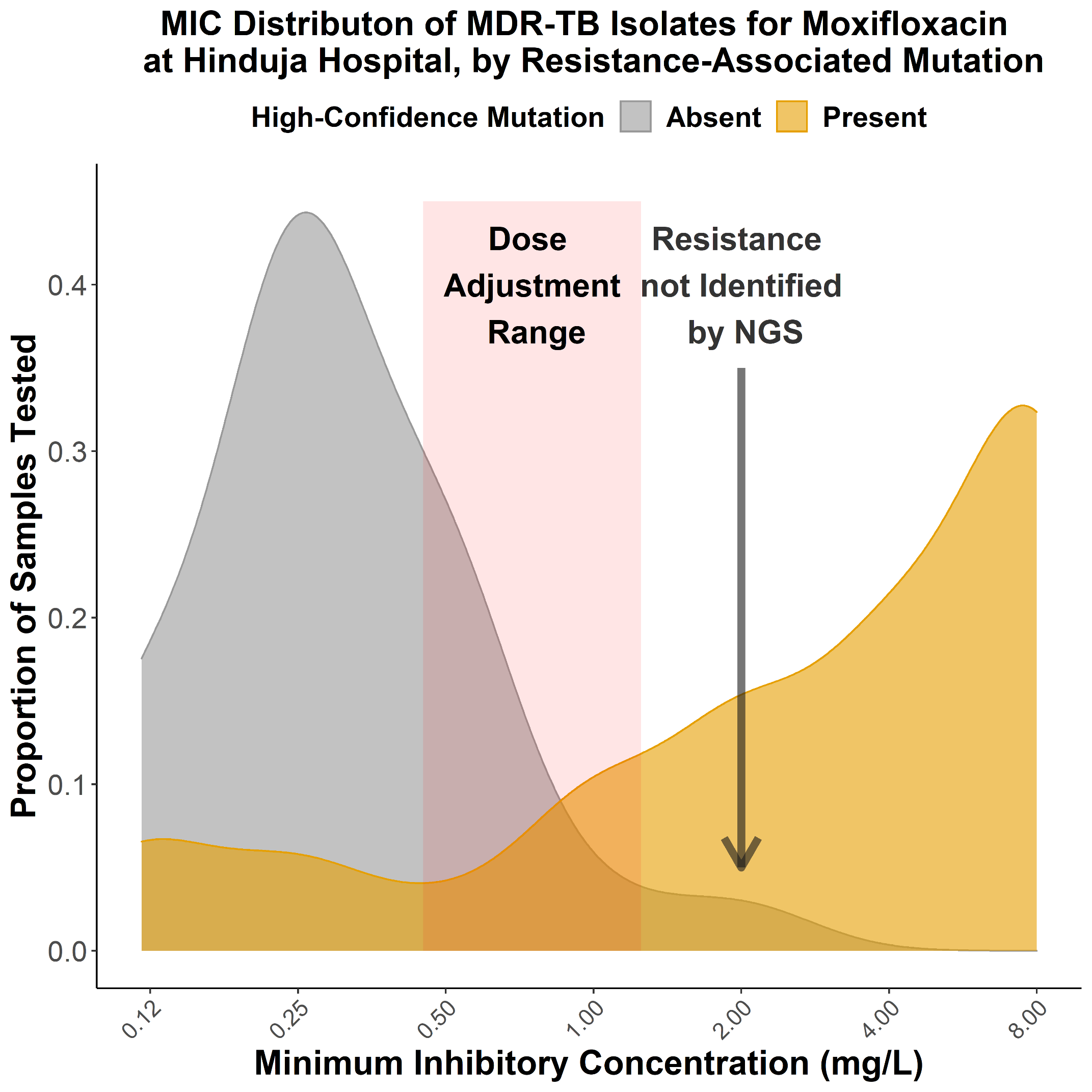
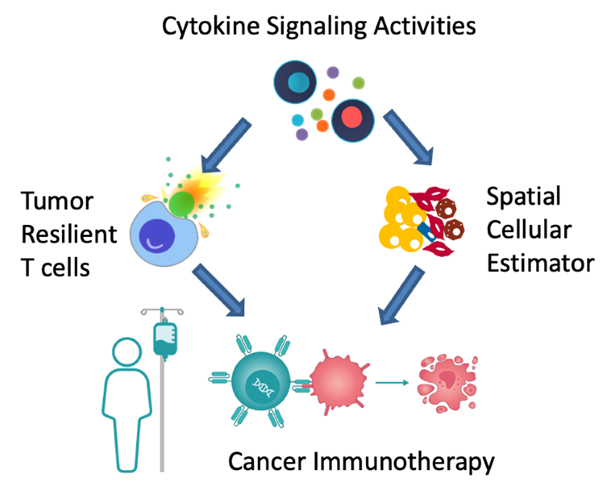
“Big Data Approaches to Study
Intercellular Signaling during
Tumor Immune Evasion”
My talk will present three computational frameworks we developed to study cytokine signaling activities and cell-cell communications during the antitumor immune response. The basic immunology tool to study cytokine signaling mostly measures cytokine release, which is transient and does not represent downstream target activities. Therefore, we first developed the CytoSig platform, providing a database of target genes modulated by cytokines and a predictive model of cytokine signaling cascades from transcriptomic profiles. We collected 20,591 transcriptome profiles for human cytokine, chemokine, and growth factor responses. This atlas of transcriptional patterns induced by cytokines enabled the reliable prediction of signaling activities in distinct cell populations in infectious diseases, chronic inflammation, and cancer using bulk and single-cell transcriptomic data. CytoSig revealed previously unidentified roles of many cytokines, such as BMP6 as an anti-inflammatory factor. Then, based on CytoSig, we developed Tres, a computational model utilizing single-cell transcriptomic data to identify signatures of T cells that are resilient to immunosuppressive signals. Tres reliably predicts clinical responses to immunotherapy in multiple cancer types using bulk T cell transcriptomic data from pre-treatment patient tumors or infusion/pre-manufacture samples for cellular immunotherapies. Further, Tres identified FIBP as a candidate immunotherapy target to potentiate adoptive cell therapy efficacies. Last, I will briefly show our SpaCET framework for deconvolving cell fractions and identifying cell-cell interactions in tumor spatial transcriptomics data.
▶RECORDING