Bio
“Reverse Engineering Chronic Mechanisms of Closed-Loop Brain Stimulation for Neuropsychiatric Disorders”
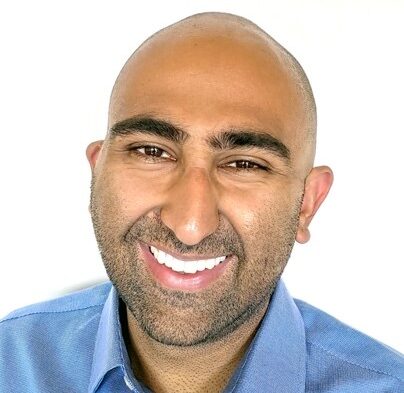
Dr. Ankit Khambhati is a biomedical engineer who specializes in the development of computational neurotechnology to map and modulate large-scale neural circuits affected by brain network disorders. He is an Assistant Professional Researcher in the Department of Neurological Surgery at the University of California, San Francisco. There he leads a research program focused on network neuromodulation and control, which integrates network science and control theory with brain electrical recordings and implantable devices to identify electrophysiologic biomarkers and develop stimulation-based strategies for rehabilitating or rewiring impaired circuits. Dr. Khambhati is currently using these techniques to investigate and optimize closed-loop brain stimulation therapy for treatment-resistant epilepsy and depression. He previously earned his B.S. in Electrical and Computer Engineering from Carnegie Mellon University and Ph.D. in Bioengineering at the University of Pennsylvania, and he completed a postdoctoral fellowship in Neuroengineering at the University of California, San Francisco.
▶RECORDING
Abstract
“Reverse Engineering Chronic Mechanisms of Closed-Loop Brain Stimulation for Neuropsychiatric Disorders”
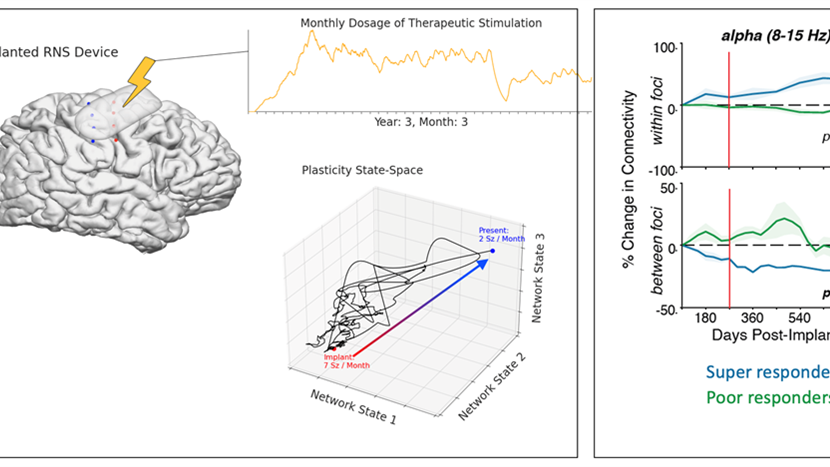
Closed-loop neuromodulation therapy that detects imminent paroxysmal events and rapidly delivers electrical stimulation to the brain using a chronically implanted device is an emerging treatment for pharmacoresistant neurologic or psychiatric disorders. Calibration of closed-loop therapy involves “expert-in-the-loop” optimization of device parameters that specify where, when, and how electrical stimulation pulses should be delivered to the brain for individual patients. A personalized stimulation strategy involves targeting discrete nodes specific to an individual’s dysfunctional brain network and triggering therapeutic stimulation based on neural biomarkers that encode the unique constellation of an individual’s symptoms. In this talk, I will present an idealized model of closed-loop stimulation therapy and its adaptation as a treatment for brain network disorders such as epilepsy and major depressive disorder. Drawing on a range of tools across engineering and neuroscience disciplines — machine learning, graph theory, stimulation-based system identification, and chronic human intracranial EEG from implanted devices – I will identify biomarkers related to naturalistic fluctuation in disease state and characterize effects of neurostimulation on functional brain network activity and connectivity. Based on these learnings, I will propose an alternate mechanism of closed-loop therapeutic efficacy based on brain network plasticity and discuss opportunities for next-generation devices.
▶RECORDING