Bio
“Mechanistic and Data-Driven Dissection of Cell Communication Through Tensor Methods”
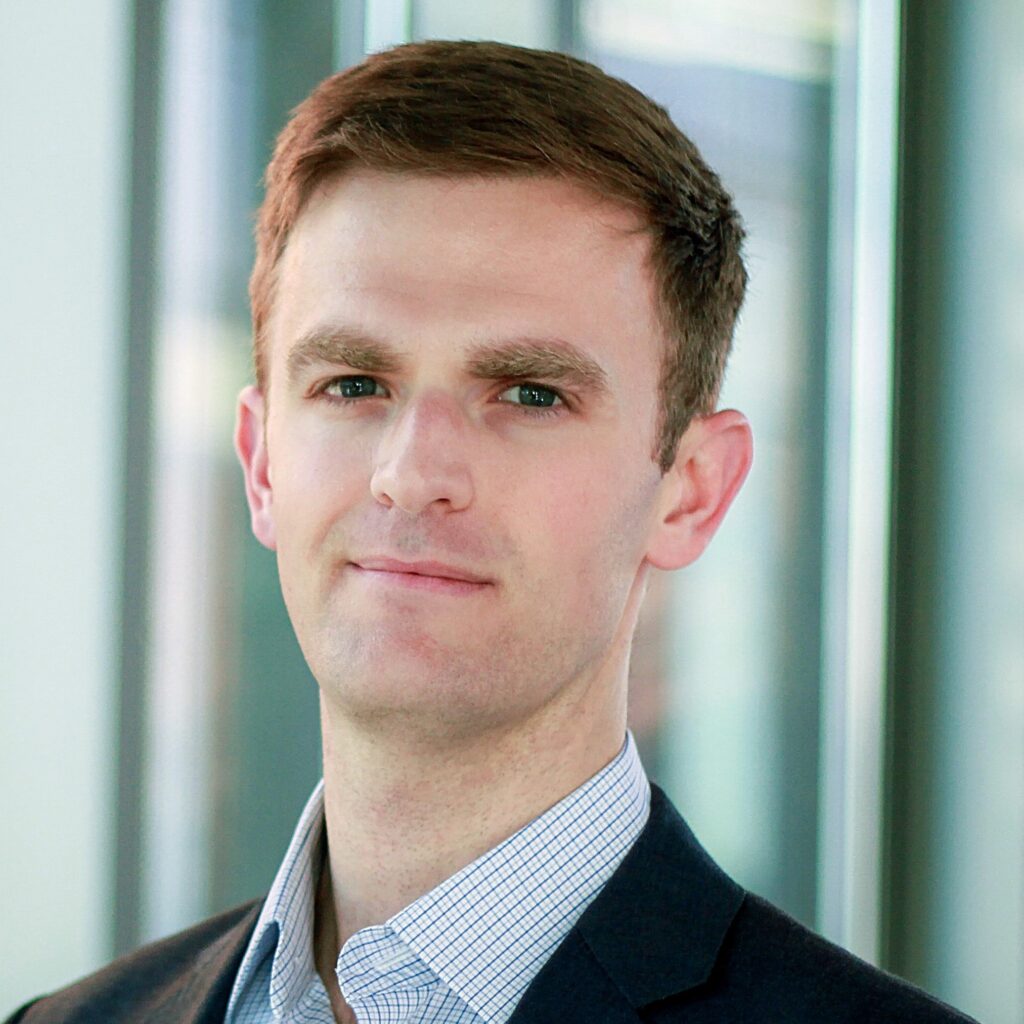
Dr. Aaron Meyer is an Assistant Professor of Bioengineering and Bioinformatics at the University of California, Los Angeles. He previously received his B.S. in Bioengineering from UCLA, his Ph.D. in Biological Engineering from the Massachusetts Institute of Technology, and then was an independent fellow at the Koch Institute for Integrative Cancer Research. Dr. Meyer’s research aims to develop new computational methods, deeply integrated with experiments, to understand intercellular communication and how it can be therapeutically manipulated. He primarily focuses on combinations of mechanistic and data-driven modeling as a solution to our incomplete knowledge of cellular pathways, and applications in cancer and immunity where models can have immediate therapeutic impact. His work has been recognized by the NIH Director’s Early Independence Award, a Siebel Scholars award, a Hellman Fellowship, the UCLA Faculty Career Development Award, the Northrop Grumman Excellence in Teaching Award, and named as Ten to Watch by the Amgen Foundation.
To join the live event please request the link by emailing: icm@jhu.edu
Recording
Abstract
“Mechanistic and Data-Driven Dissection of Cell Communication Through Tensor Methods”

Studies of even simple cell responses to their environment are hindered by how responses are multi-dimensional. For example, a simple receptor-ligand pathway can display differing responses based on timescale, cell type, stimulation, type of response measured, and context. Interrogating and manipulating these systems is thus almost always constrained by an incomplete view of the overall pathway.
Like how principal component analysis uses a low-rank approximation for dimensionality reduction of matrix-structured data, tensor generalizations provide solutions for pattern recognition in data with a higher-dimensional structure. Using several recent and unpublished applications, including engineering cell-type selective IL-2 therapies, serology analysis, and clinical multi-omic studies of infection response, I will describe some of the unique benefits of tensor-based analysis and the biological discoveries it has revealed. Specifically, tensor approximations enable more effective dimensionality reduction, separation of dimension-specific effects, and a natural, flexible solution to data integration. Finally, I will discuss some of the reasons tensor-based methods remain limited in their application to molecular biology. Resolving these limitations, and applying tensor methods in a more widespread manner, will help provide a complete view of cellular communication.
Recording