Bio
“Interaction-Based Methods to Uncover Cancer Genes”
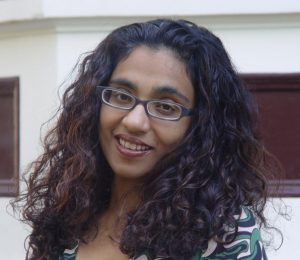
Dr. Mona Singh obtained her AB and SM degrees at Harvard University, and her PhD at MIT, all three in Computer Science. She did postdoctoral work at the Whitehead Institute for Biomedical Research. She has been on the faculty at Princeton since 1999, and currently she is Professor of Computer Science in the computer science department and the Lewis-Sigler Institute for Integrative Genomics. Her group works broadly in computational molecular biology, as well as its interface with machine learning and algorithms. Her group is especially interested in developing methods for predicting and characterizing protein specificity, interactions, and networks. She is a Methods Editor at PLOS Computational Biology, has been program committee chair for several major computational biology conferences, including ISMB (2010), WABI (2010), ACM-BCB (2012), and RECOMB (2016), and has been Chair of the NIH Modeling and Analysis of Biological Systems Study Section (2012-2014). She received the Presidential Early Career Award for Scientists and Engineers (PECASE) in 2001, and is an ISCB Fellow.
Click here to view webcast.
Abstract
“Interaction-Based Methods to Uncover Cancer Genes”
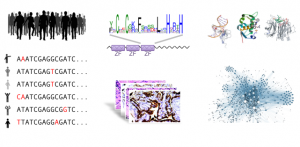
A major aim of cancer genomics is to pinpoint which somatically mutated genes are involved in tumor initiation and progression. This is a difficult task, as numerous somatic mutations are typically observed in each cancer genome, only a subset of which are cancer-relevant, and very few genes are found to be somatically mutated across large numbers of individuals. In this talk, I will demonstrate that molecular interactions and networks provide a powerful context within which to interpret cancer mutational data. First, I will introduce an analytical framework to identify genes whose interaction or other functional properties are preferentially perturbed in cancer genomes. Next, I will show how to leverage per-individual mutational profiles within the context of protein-protein interaction networks in order to identify small connected subnetworks of genes that, while not individually frequently mutated, comprise pathways that are altered across (i.e., “cover”) a large fraction of individuals. Overall, these methods recapitulate known cancer driver genes, and discover novel, and sometimes rarely-mutated, genes with likely roles in cancer.
Click here to view webcast.